Improving DNA Barcode-based Fish Identification System on Imbalanced Data using SMOTE
Abstract: Problem in
imbalanced data is very common in classification or identification. The problem
is raised when the number of instances of one class far exceeds the other. In
the previous research, ourDNA barcode-based Identification System of Tuna and
Mackerel was developed in imbalanced dataset.The number of samples of Tuna and
Mackerel were much more than those of other fish samplesTherefore, the accuracy
of the classification model was probably still in bias. This research aimed at employing
Synthetic Minority Oversampling Technique (SMOTE) to yield balanced dataset. We
used kmers frequencies from DNA barcode sequences as features and Support
Vector Machine (SVM) as classification method. In this research we used
trinucleotide (3-mers) and tetranucleotide (4-mers). Thetraining dataset was
taken from Barcode of Life Database (BOLD). For evaluating the model, we
compared the accuracy of model using SMOTE and without SMOTE in order to
classify DNA barcode sequences which is taken from Department of Aquatic
Product Technology, Bogor Agricultural University. The results showed that the
accuracy of the model in the species level using SMOTE was 7% and 13% higher
than those of non-SMOTE for trinucleotide (3-mers) and tetranucleotide
(4-mers), respectively. It is expected that the use of SMOTE, as one of data
balancing technique, could increase the accuracy of DNA barcode based fish
classification system, particularly in the species level which is difficult to
be identified.
Author: Wisnu Ananta Kusuma
Journal Code: jptkomputergg170031
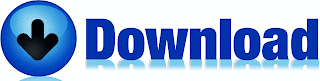