ONLINE NEWS CLASSIFICATION USING NAÏVE BAYES CLASSIFIER WITH MUTUAL INFORMATION FOR FEATURE SELECTION
Abstract: The number of online
news documents can reach billion documents. Therefore, the grouping of news
documents required to facilitate a editorial staff to input and categorize news
by its categories. This paper aim to classify online news using Naive Bayes
Classifier with Mutual Information for feature selection that aims to determine
the accuracy from combination of this methods in the classification of online
news documents, so grouping of online news documents can be classified automatically
and achieve more accurate for classification model. Data is divided into
training and testing data. Data in August, September and October 2016 was used
for training data. For testing data, 65 documents was used that located in
November. The best results of this methods are 80% of accuracy, 94.28% of
precision, 79.68% of recall and 85.08% of f-measure for Multivariate Bernoulli
without feature selection. Then the best results of classification model using
Mutual Information for feature selection achieved in Multivariate Bernoulli
model with 70% of accuracy, 89.11% of precision, 69.76% of recall and 78.04% of
f-measure with the word’s efficiency rate until 52% than before using feature
selection. In other hand, the results of Multinomial Naïve Bayes without
feature selection are 41.67% of accuracy, 75.68% of precision, 41.90% of recall
and 48.13% of f-measure, for the results of Multinomial Naïve Bayes model using
feature selection are 10% of accuracy, 33.33% of precision, 9.40% of recall and
14.35% of f-measure.
Keywords: Classification,
Feature Selection, Multinomial Naïve Bayes, Multivariate Bernoulli, Mutual
Information, Naïve Bayes Classifier
Penulis: Shafrian Adhi Karunia
Kode Jurnal: jptinformatikadd170129
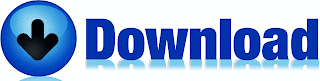