Metode Jaringan Syaraf Tiruan Untuk Prediksi Performa Mahasiswa Pada Pembelajaran Berbasis Problem Based Learning (PBL)
Abstrak: In order to improve
academic quality in higher education, students’ performance evaluation is
becoming important. To prevent increasing failure rate in the course, we need a
system that is capable of predicting student’s performance in the end of the
course. The research used several factors that are considered to affect
students' performance on Problem Based Learning (PBL), such as students’
demography, students’ prior knowledge and group heterogeneity. The method used in the study was Artificial
Neural Network (ANN) with backpropagation training algorithm. Total 8 neurons
were used as inputs for ANN which were obtained from gender variable (2
neurons), age variable (1 neuron), students’ average knowledge variable (1
neuron), students’ average skill variable (1 neuron) and group heterogeneity
variable (3 neurons). Several different ANN architecture were tested in the
study using 2, 7 and 12 hidden neurons respectively. Each architecture was
trained using various different training parameters in order to find the best
ANN architecture. Dataset used in the
research were obtained from Academic Information System in Faculty of Dentistry
Unissula which contained Adult and Elderly Diseases Course’s participants from
year 2009 to 2013. The ANN output were numeric values which represented
students’ performance in Adult and Elderly Diseases Course. The output of this
study is a system that is able to predict the student performance in block
course. The result shows that using 7 hidden neurons in the network combining
with 0.5 ,0.1 and 9000 for learning
rate, momentum and epoch respectively, were the best ANN architechture and
parameters in the study. The MSE obtained from validation test was 0,011926
with correlation coefficient (R) 0,796879. The prediction system are expected
to help faculty and academic evaluation team to conduct actions to improve
student’s academic performance and prevent them from failure in the course.
Penulis: Badieah, Rachmat
Gernowo, Bayu Surarso
Kode Jurnal: jptinformatikadd160406
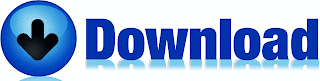