Comparing performances of clements, box-cox, Johnson methods with weibull distributions for assessing process capability
Abstract: This study examines
Clements’ Approach (CA), Box-Cox transformation (BCT), and Johnson
transformation (JT) methods for process capability assessments through
Weibull-distributed data with different parameters to figure out the effects of
the tail behaviours on process capability and compares their estimation
performances in terms of accuracy and precision.
Design/methodology/approach: Usage of process performance index (PPI) Ppu
is handled for process capability analysis (PCA) because the comparison issues
are performed through generating Weibull data without subgroups. Box plots,
descriptive statistics, the root-mean-square deviation (RMSD), which is used as
a measure of error, and a radar chart are utilized all together for evaluating
the performances of the methods. In addition, the bias of the estimated values
is important as the efficiency measured by the mean square error. In this
regard, Relative Bias (RB) and the Relative Root Mean Square Error (RRMSE) are
also considered.
Findings: The results reveal that the performance of a method is
dependent on its capability to fit the tail behavior of the Weibull
distribution and on targeted values of the PPIs. It is observed that the effect
of tail behavior is more significant when the process is more capable.
Research limitations/implications: Some other methods such as Weighted
Variance method, which also give good results, were also conducted. However, we
later realized that it would be confusing in terms of comparison issues between
the methods for consistent interpretations.
Practical implications: Weibull distribution covers a wide class of
non-normal processes due to its capability to yield a variety of distinct
curves based on its parameters. Weibull distributions are known to have
significantly different tail behaviors, which greatly affects the process
capability. In quality and reliability applications, they are widely used for
the analyses of failure data in order to understand how items are failing or
failures being occurred. Many academicians prefer the estimation of long term
variation for process capability calculations although Process Capability
Indices (PCIs) Cp and Cpk are widely used in literature. On the other hand, in
industry, especially in automotive industry, the PPIs Pp and Ppk are used for
the second type of estimations.
Originality/value: Performance comparisons are performed through
generating Weibull data without subgroups and for this reason, process
performance indices (PPIs) are executed for computing process capability rather
than process capability indices (PCIs). Box plots, descriptive statistics, the
root-mean-square deviation (RMSD), which is used as a measure of error, and a
radar chart are utilized all together for evaluating the performances of the
methods. In addition, the bias of the estimated values is important as the
efficiency measured by the mean square error. In this regard, Relative Bias
(RB) and the Relative Root Mean Square Error (RRMSE) are also considered. To
the best of our knowledge, all these issues including of execution of PPIs are
performed all together for the first time in the literature.
Keywords: Process performance
indices (PPIs), process capability indices (PCIs), process capability analysis
(PCA), non-normal processes
Author: Ozlem Senvar, Bahar
Sennaroglu
Journal Code: jptindustrigg160023
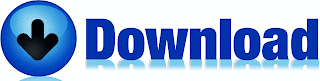